- Thread starter
- #1
Omar 382
Well-Known Member
Most people understand, or at least believe, that a run differential of about 10 runs leads to 1 win. I used a linear regression, and got the following output:
Therefore, a team's estimated winning percentage can be obtained from the following formula:
Wpct = 0.4999918 + 0.0006287 × RD
This formula tells us that a team with a run differential of 0, or 750 runs allowed and 750 runs scored, can expect to win about half its games, or 81 games. In addition, a one unit increase in run differential leads to a 0.0006287 increase in winning percentage. Therefore, a team scoring 760 runs and allowing 750 has a run differential of +10 and is predicted to have a winning percentage of 0.500+10·0.0006287 ≈ 0.506. A .506 winning percentage in a 162 game season corresponds to about 82 wins.
I analyzed all teams since 2000, and plotted their residuals (basically the difference between the actual and estimated winning percentages of each team) versus the run differential for the fitted linear model. Here are my results:
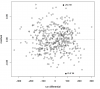
The graphic may make the model not appear as effective as it should be, as there are quite a few points away from the straight line, but you must remember that I used -0.05 and 0.05 as parameters. If I instead used -0.10 and 0.10, the dots would appear even closer to the line.
[If you are wondering about the model's efficacy, read this, if not; then don't. I took the root mean square error, abbreviated as RMSE, to estimate the average magnitude of the errors. Approximately two thirds of the residuals fall between −RMSE and +RMSE, while 95% of the residuals are between −2·RMSE and 2·RMSE. Therefore, my model looks fairly sound.]
The funny thing I noticed were the two outliers: the 2008 Angels and the 2006 Indians. The Angels had a +68 run differential, they were supposed, according to the linear equation, to have a 0.542 winning percentage; they ended the season at 0.617. The residual value for this team is 0.617−0.542 = 0.075. On the other side, the 2006 Cleveland Indians, with a +88 run differential, are seen as a 0.555 team by the linear model, but they actually finished at a mere 0.481, corresponding to the residual 0.481 − 0.555 = −0.073.
I wonder what, if anything observable, caused these two teams to over and underperform the linear model. Questions and comments are welcome!
Therefore, a team's estimated winning percentage can be obtained from the following formula:
Wpct = 0.4999918 + 0.0006287 × RD
This formula tells us that a team with a run differential of 0, or 750 runs allowed and 750 runs scored, can expect to win about half its games, or 81 games. In addition, a one unit increase in run differential leads to a 0.0006287 increase in winning percentage. Therefore, a team scoring 760 runs and allowing 750 has a run differential of +10 and is predicted to have a winning percentage of 0.500+10·0.0006287 ≈ 0.506. A .506 winning percentage in a 162 game season corresponds to about 82 wins.
I analyzed all teams since 2000, and plotted their residuals (basically the difference between the actual and estimated winning percentages of each team) versus the run differential for the fitted linear model. Here are my results:
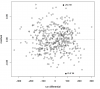
The graphic may make the model not appear as effective as it should be, as there are quite a few points away from the straight line, but you must remember that I used -0.05 and 0.05 as parameters. If I instead used -0.10 and 0.10, the dots would appear even closer to the line.
[If you are wondering about the model's efficacy, read this, if not; then don't. I took the root mean square error, abbreviated as RMSE, to estimate the average magnitude of the errors. Approximately two thirds of the residuals fall between −RMSE and +RMSE, while 95% of the residuals are between −2·RMSE and 2·RMSE. Therefore, my model looks fairly sound.]
The funny thing I noticed were the two outliers: the 2008 Angels and the 2006 Indians. The Angels had a +68 run differential, they were supposed, according to the linear equation, to have a 0.542 winning percentage; they ended the season at 0.617. The residual value for this team is 0.617−0.542 = 0.075. On the other side, the 2006 Cleveland Indians, with a +88 run differential, are seen as a 0.555 team by the linear model, but they actually finished at a mere 0.481, corresponding to the residual 0.481 − 0.555 = −0.073.
I wonder what, if anything observable, caused these two teams to over and underperform the linear model. Questions and comments are welcome!